
In a regression model, the residual variance is defined as the sum of squared differences between predicted data points and observed data points. This tells us that the residual variance in the ANOVA model is high relative to the variation that the model actually can explain. This means we don’t have sufficient evidence to say that the mean difference between the groups we’re comparing is significantly different. 05, we do not have sufficient evidence to reject the null hypothesis. The F-value in the ANOVA table above is 2.357 and the corresponding p-value is 0.113848. To determine if this residual variance is “high” we can calculate the mean sum of squared for within groups and mean sum of squared for between groups and find the ratio between the two, which results in the overall F-value in the ANOVA table. In the ANOVA model above we see that the residual variance is 1,100.6. This value is also referred to as “sum of squared errors” and is calculated using the following formula: The value for the residual variance of the ANOVA model can be found in the SS (“sum of squares”) column for the Within Groups variation. Whenever we fit an ANOVA (“analysis of variance”) model, we end up with an ANOVA table that looks like the following:
Residual statistics how to#
The following examples show how to interpret residual variance in each of these methods. Regression: Used to quantify the relationship between one or more predictor variables and a response variable. ANOVA: Used to compare the means of three or more independent groups.Ģ. Residual variance appears in the output of two different statistical models:ġ. The higher the residual variance of a model, the less the model is able to explain the variation in the data.
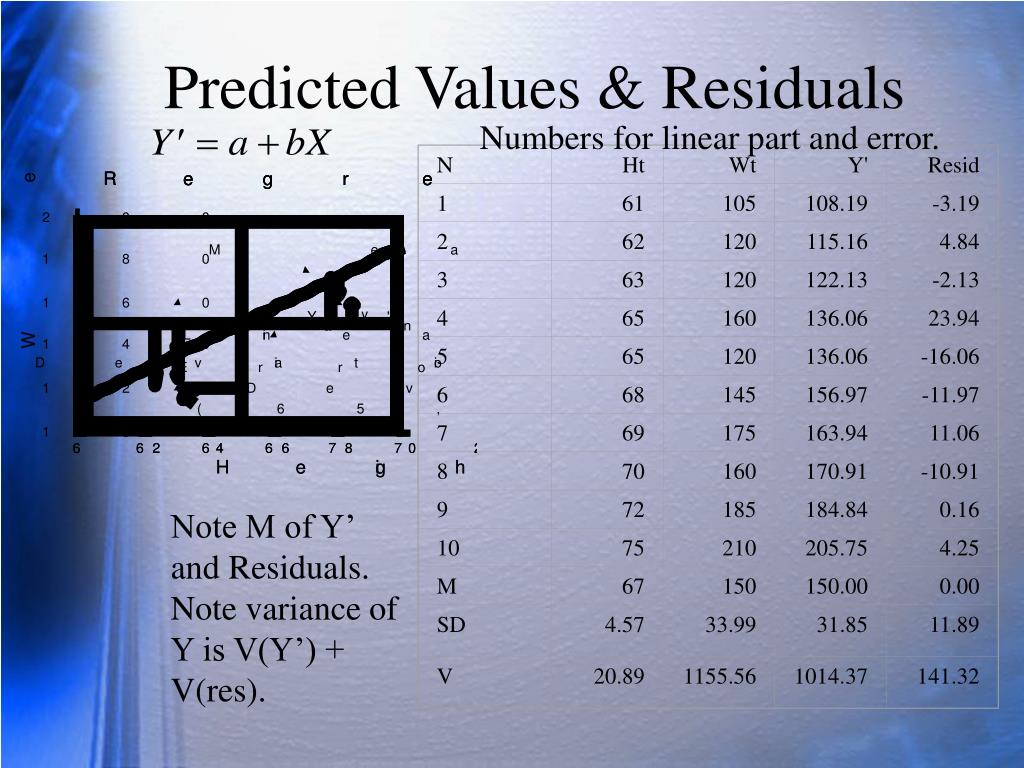
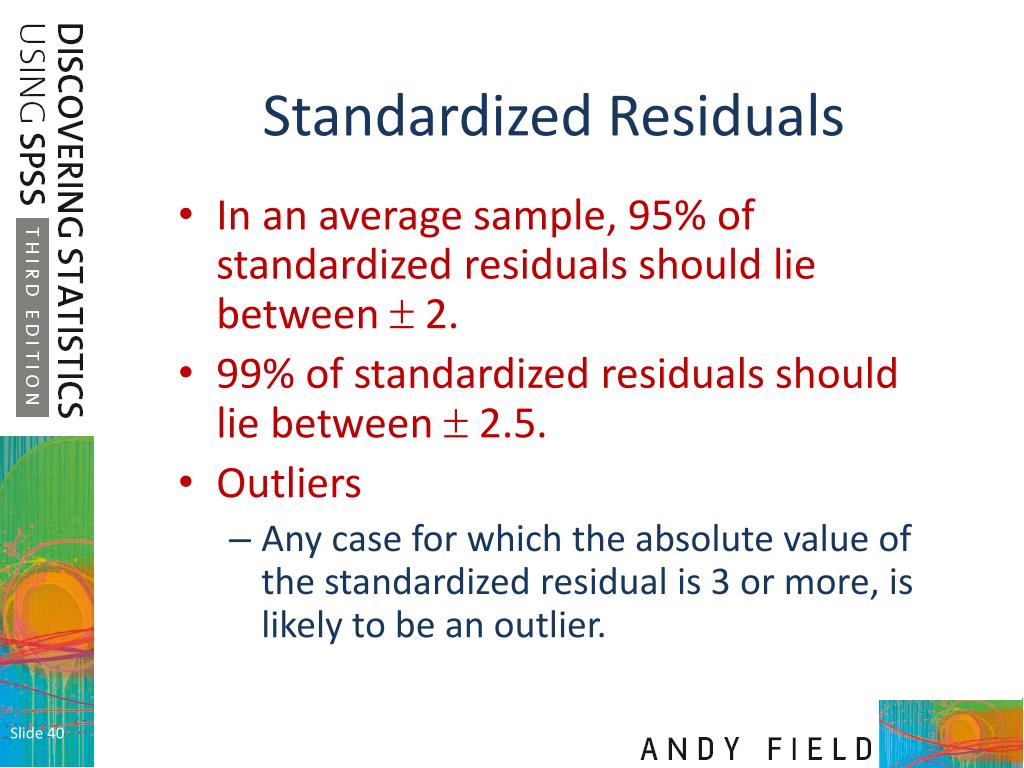
Again, the best option for a duration response should be survival analysis.Residual variance (sometimes called “unexplained variance”) refers to the variance in a model that cannot be explained by the variables in the model. You can compare this random-effect specification with your current crossed clustering by a likelihood ratio test, using anova() with estimation by REML, varCompTest() in. Therefore, a tentative random effect specification might be (0 + borrower.Gender + log(borrowing.Amount) | interaction(borrower.Country, borrower.Sector)), where 0 + borrower.Gender moves random intercepts to around the gender indicator, so the standard deviation of random intercepts differ by gender. And there might be a random slope if the effect of loan amount on time to loan deviates by country-sector pair, and this random slope might be correlated with the random intercept. However, this within-country and within-sector variability might differ by gender. The intercept has random effects in your current model specification. Further, variables with random effects also need investigation. Perhaps clustering by country-specific sector alone is better, which requires coding each country-sector pair as a unique group. The clustering structure also needs attention, as I don't think that Sector S in Country A is comparable with Sector S in Country B to share the same random effects of sector. Check the fixed-effect predictor significance by anova() with estimation by ML. Add additional predictors may substantially improve the residual diagnosis results. Coefficients in a fixed-effect specification in the form of log(y) ~ log(x) measures elasticity directly.
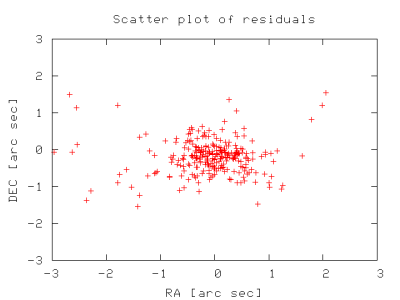
Try at least logarithm, quadratic and cubic terms of loan amount and interaction terms between gender and loan amount to remove part of the nonnormality and heterogeneity in the error term. You should make a plot between each response-predictor pair to help determine the function form. In addition to a limited range of the dependent variable, these violations can be caused by other peculiarity, such as the functional form of predictors. This is because the standard errors of each fixed-effect coefficient is biased, despite its consistency if the number of groups (country and sector in your case) and the number of customers clustered in each group are large. The point estimates of fixed effects' coefficients and predicted random effects are still unbiased. This affects inference but not point estimates of the model: The p-value and confidence intervals of each coefficient, as well as confidence and prediction intervals of predicted means, are doubtful. The residual plots reflect that the assumptions of residual normality and homogeneity are violated. What do these two plots mean? Does the same set of assumptions (normality of residuals homogenity of variance) apply for linear mixed effects model? Am I right in reading that this model is not properly specified as it violates normality assumptions?
